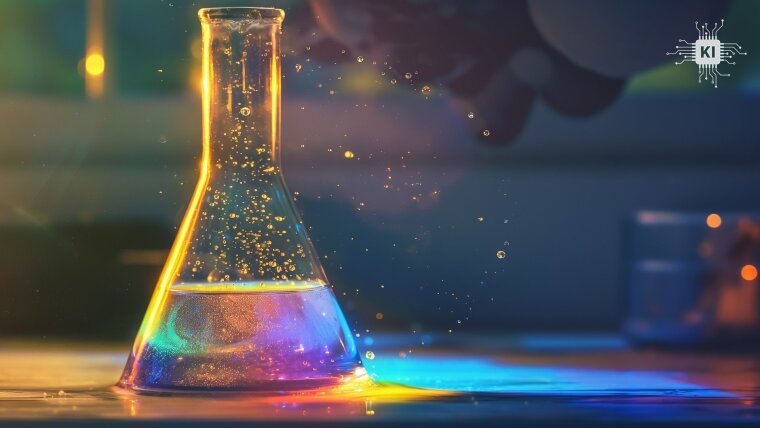
By Marco Körner
GPT-3, the language model behind the renowned AI-based tool ChatGPT, can also be used in the field of chemistry to perform a range of research tasks. Its application has been demonstrated by researchers from the École polytechnique fédérale de Lausanne (EPFL), the University of Jena and the Helmholtz Institute for Polymers in Energy Applications (HIPOLE) Jena. As the team reported in a recent article in »Nature Machine Intelligence«, they have successfully circumvented a common problem in chemistry: the lack of high volumes of data needed to train AI.
Curated Q&As replace vast pools of data
»One of the various examples we used was so-called photosensitive switches,« explains Kevin Jablonka, lead author of the article. »These are molecules that change their structure when exposed to light of a certain wavelength. They also occur in the human body: our retinal cells contain a molecule called rhodopsin, which reacts to light and thus ultimately serves as the chemical switch that converts optical signals into nerve impulses,« he adds.
»The question of whether and how a hitherto unknown molecule can be switched by light is highly relevant, for instance when it comes to developing sensors, « he summarizes. »But we’ve also incorporated the question of whether a molecule can be dissolved in water, « Jablonka mentions another example. »Solubility in water is an important factor for pharmacological agents to exert their desired effect in the body.«
In order to train their GPT model to tackle these and other questions, however, the research group had to resolve a fundamental problem: »GPT-3 is not familiar with the vast majority of specialist chemical literature,« explains Jablonka. »As a result, the answers we can generate from this model are usually limited to what’s available on Wikipedia.«
With this in mind, the research group adopted a more targeted approach, enhancing GPT-3 with a comparatively small dataset of questions and answers. »We fed the model with questions—for example, about photosensitive switchable molecules, but also regarding the water solubility of certain molecules and other chemical aspects—and completed its training by providing the known answer to each question,« explains Jablonka. Proceeding in this way, he and his team created a language model capable of providing accurate insights into a variety of chemical queries.
Finally, they began to test the model. »The scientific question about a light-switchable molecule might look like this: What is the pi→pi* transition of CN1C(/N=N/ C2=CC=CC=C2)=C(C)C=C1C?« Jablonka says. He explains that, because the model is text-based, structural formulae cannot be used as inputs. »However, our GPT is able to handle so-called SMILES codes for molecules, like in this example.«. The tool is also capable of processing other notation methods, including chemical names following IUPAC nomenclature.
As easy as a literature search
During the testing phase, the model solved all manner of chemical problems. In many cases, it actually fared better than similar models developed to date by researchers using vast quantities of data. »The decisive factor, however, is that our GPT is as easy to use as a literature search function and works for an array of chemical problems—from substance properties such as solubility to thermodynamic and photochemical properties such as solution enthalpy and interaction with light and, of course, chemical reactivity,« adds Prof. Dr Berend Smit of EPFL Lausanne.