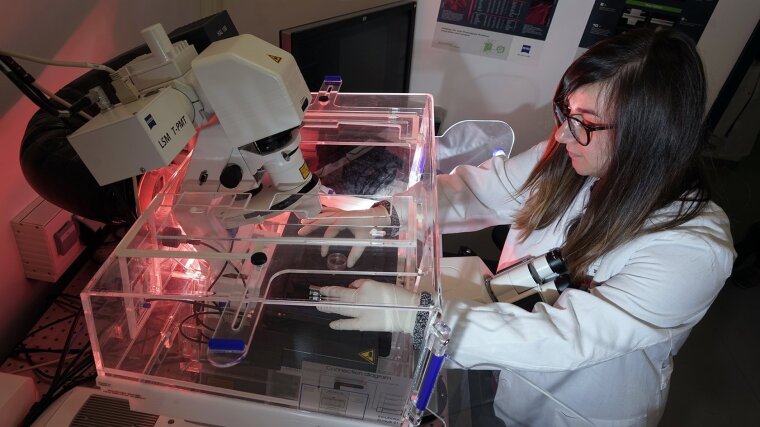
By Ute Schönfelder
The research group for Applied Systems Biology led by Prof. Dr Marc Thilo Figge applies AI methods in their biomedical research. Based at the Institute of Microbiology and the Leibniz Institute for Natural Product Research and Infection Biology (Leibniz-HKI), the team hopes that this will enable them to identify infection processes caused by human-pathogenic fungi faster and more precisely.
Fungal infections pose a potentially life-threatening risk, especially for people with a weakened immune system. Most of these infections are caused by mould-like fungi such as Aspergillus fumigatus and yeast fungi such as Candida albicans. These microorganisms are practically ubiquitous in the world around us and also appear in the human body. A properly functioning immune system keeps their growth at bay, meaning that the human body generally keeps these fungi well in check. However, if a person’s immune defences are weakened, perhaps due to an illness or because they take immunosuppressants, the pathogens can spread and trigger life-threatening sepsis.
Prof. Figge explains the problem to date: »Diagnosing blood infections, such as those caused by fungi within the Candida genus, is a difficult and protracted process, which reduces the patients’ treatment options and, ultimately, their chance of survival.« When it comes to invasive fungal infections, he says, precise—and, above all, swift—diagnosis can quite literally save lives.
This is where AI methods come in. Researchers are applying artificial neural networks to analyse both microscopic images and video data of whole blood samples. Although it is not possible to directly observe the pathogens, which occur in low numbers in the patients’ blood, it is possible to detect their interactions with blood cells, which appear in high numbers. Neutrophils, for example, form part of the body’s immune system and can be isolated from the whole blood. They demonstrate a characteristic, dynamic change in their outer form following contact with fungal pathogens.
The morphodynamics of a neutrophil following infection with Candida in whole blood. These cells demonstrate dynamic changes in their morphology following contact with Candida pathogens. There are characteristic morphodynamic differences for different fungi, such as Candida albicans and Candida glabrata which can be used to differentiate the pathogens of a blood infection by means of AI. These images show the morphological change of the same neutrophil at different points in a two-minute period (series runs from left to right)
Picture: AG Figge»This changing appearance over time, which we call the morphodynamics of neutrophils, is an indicator of infection,« explains Figge. Analyses that have been conducted to date by conventional means—that is, with the human eye—can now be carried out with the assistance of AI tools. Figge says that whole blood samples with no pathogens present can be distinguished from those with pathogens with 100% accuracy. But that’s not all: »The AI can even reliably distinguish between two closely related fungal pathogens, such as Candida albicans and Candida glabrata.« Not only does the technology work more quickly than a human, it also delivers more precise results.
The team deploys different types of neural networks, using convolutional neural networks (CNNs) to analyse individual microscopic images and transformer neural networks to examine video data. Both models are trained using neutrophils isolated from whole blood.
»Inherently opaque black boxes«
Despite the considerable successes in applying AI methods in this area to date, Prof. Figge and his research team also look critically at artificial neural networks and their growing use. »The fact that these tools are inherently opaque black boxes leaves behind an unsatisfying aftertaste,« he says. Any insights into the characteristics upon which the decision-making processes in artificial neural networks are based remain concealed. »This lack of transparency is cause for concern, especially in critical biomedical research applications where AI-based decisions in diagnostic settings influence the treatment people receive.«
In the future, Figge argues, researchers must devote greater attention to developing methods that enable us to interpret AI methods more effectively. This means finding a balance between the complexity of advanced algorithms and the need for transparency and accountability in decision-making processes.