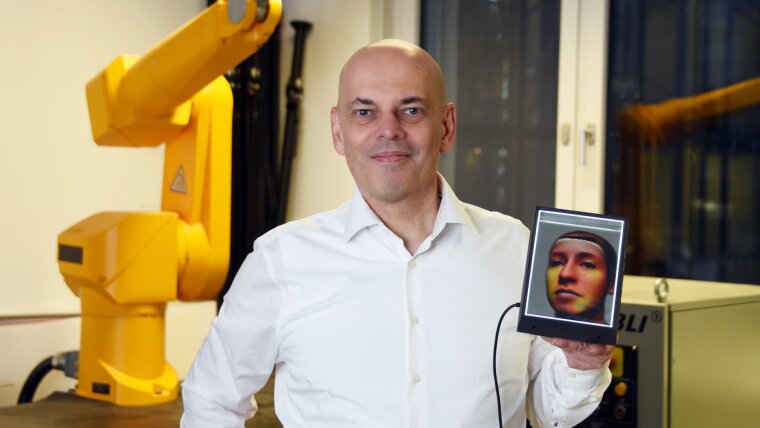
Artificial intelligence, usually referred to simply as AI, is the topic on everyone’s lips. Growing numbers of people are now aware of AI, certainly since generative AI tools like ChatGPT were made available for anyone to use. Nevertheless, AI has long been integrated in many everyday applications, from voice assistants to image recognition tools to apps that offer book and film recommendations tailored to your personal tastes. But just how »intelligent« is AI? What is it capable of? And what is—as of yet—beyond its abilities? We explored these issues in an interview with computer scientist and AI development expert Prof. Dr Joachim Denzler.
Interview by Ute Schönfelder
What exactly does AI mean?
The term »artificial intelligence« is already quite old. It was coined in the 1950s and originally referred to the notion of developing machines that could act like humans, with the same capabilities as humans. As we know, such machines still do not exist. What’s more, they are unlikely to exist any time soon.
Despite this, we find ourselves talking about AI more than ever.
Yes, but we use the term to refer to something else. Based on the original idea of emulating humans, researchers in the 1960s began recreating the basic structures of the human brain, creating artificial neural networks. While this approach yielded considerable progress—in pattern recognition and machine learning, for example—it did not come close to genuine AI.
We now have so-called »deep neural networks« that perform »deep learning« and are very powerful. These networks have now become synonymous with AI in common parlance.
But are these networks »genuine« AI?
No; that’s why we refer to them as »weak AI«. In truth, this term covers all currently available AI tools and technologies, from statistical data analysis, machine learning and deep learning to autonomous vehicles and voice assistants.
These are all machines designed to perform defined tasks and which are only suitable for these tasks. Nowadays, we’re able to integrate increasing numbers of tasks into these systems, and their processes run very swiftly and efficiently, which can create the illusion of real intelligence. Ultimately, however, we are still working in the field of machine learning and, therefore, remain in the field of weak AI.
What would »strong« AI look like? And when will we be able to use it?
In contrast to the weak AI tools I’ve described, which are used for specific tasks, strong AI is universally applicable. It can solve almost any problem at least as well as a human could. This means it can act independently, flexibly, and proactively. However, we will likely have to wait some time yet before we have this »strong AI«.
How are researchers trying to make this a reality?
One current avenue of exploration is the development of AI models trained with very different modalities. Just as we humans have different sensory organs that »feed« our brain with different stimuli, we are now attempting to train AI with different text, image and sensor data to convey increasingly complex »knowledge«. However, strong AI would require the machine to be able to acquire knowledge independently before transferring that knowledge and applying it to other areas.
Such models would bring about significant advances. But does this represent the step to strong AI? I don’t think so.
To what extent do you engange with AI in your own work?
My team and I develop our own artificial neural networks. One topic we’re working on at present is known as causal inference. In other words, we’re trying to teach the machines the principle of cause and effect.
So, instead of merely identifying statistical relationships in datasets, AI should determine the cause of the correlation between them. For example, there is a correlation between ice cream sales and the use of air-conditioning systems. The underlying cause is the outdoor temperature.
How are you proceeding here?
To begin with, it looks exactly like AI has functioned to date: we train AI models with known principles of cause and effect from different areas of application. We use hybrid modelling approaches like this in our work at the ELLIS Unit (Combating the climate crisis with AI).
Later on, however, the machines should be able to distinguish between correlation and causation themselves. As humans, we usually determine this by intervening in a system. If, for example, I want to know whether it’s warm in my office because I’ve turned the heating up or because the sun is shining through the window, I can find out by switching off the heating and checking whether the room temperature changes. Alternatively, I can pull down the blind and see what happens.
In order to teach neural networks to understand cause and effect, we use time-series data, such as water levels in rivers. These levels depend on the water levels in tributaries, among other factors. We try to train the model to understand this relationship by changing the time series. This way, the model learns whether one variable influences another.
What do you consider to be the challenges and limitations in the use of AI?
In addition to technological implementation, which I mentioned earlier, I think the vast energy requirements of these systems pose a further challenge. The current computing paradigm, in which systems have to become bigger in order to become more powerful, means that their energy consumption is rising immeasurably. Given the finite resources at our disposal and the challenge of climate change, this is a problem we absolutely must solve.
The ethical issues of AI are an additional aspect. Current AI models are already so complex that no human can fully understand them. This raises the issue of the credibility of the results produced by an AI model and how we should deal with them, in a medical setting, for example, in relation to diagnoses and possible treatment options.
AI, however, has also already been applied in some legal proceedings. In the USA, AI models have been used to assess the likelihood that criminals will re-offend. People are assessed differently depending on their ethnicity—and such cases are incredibly delicate from an ethical perspective.
Is there anything you think AI will never be able to do?
I’d rather not make any predictions about that. If you’d asked me that ten years ago, I wouldn’t even have thought it was possible to achieve what is already possible today. I don’t think we can even imagine the developments that will emerge in the years ahead. That’s why I’m very cautious when making predictions like that.
What AI tool or AI-based technology do you personally hope to see?
In truth, I’d like to see what AI was originally meant to be: a sort of personal assistant, a machine that can help me with all my day-to-day tasks. That would be useful.